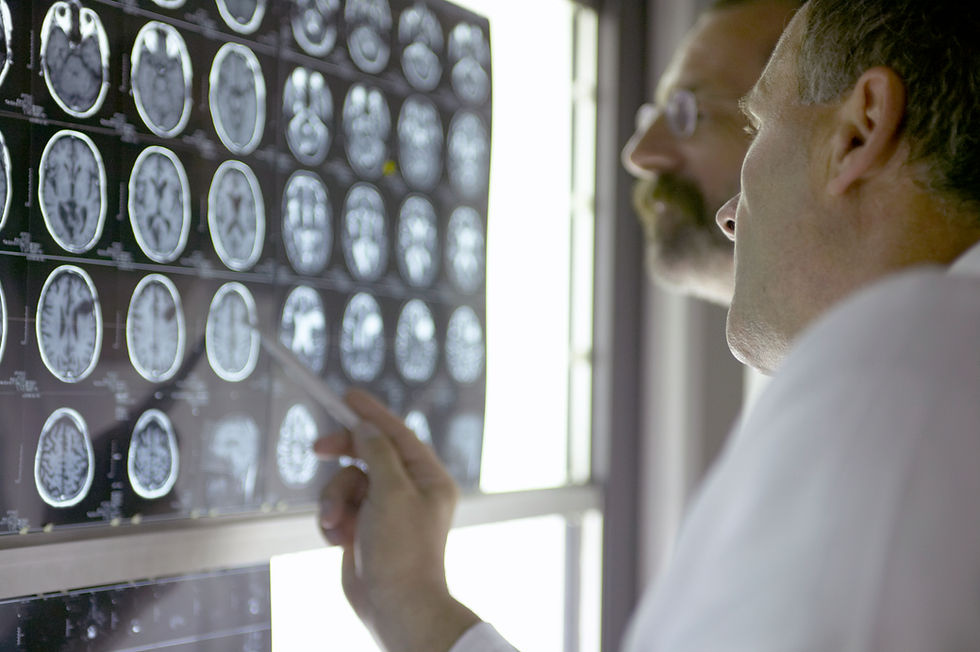
Predictive analytics in patient recruitment and retention is a valuable approach used in clinical trials to improve the process of enrolling participants and ensuring their continued engagement throughout the study. It involves using historical data, various patient-related factors, and statistical modeling to predict which individuals are most likely to enroll in a clinical trial and remain in the study until its completion. Here's an explanation of patient recruitment and retention predictive analytics:
Patient Recruitment Predictive Analytics: 1. Historical Data Analysis: The process begins by analyzing historical trial data, which includes information about previous trial participants, such as demographics, medical history, geographic location, and recruitment sources. 2. Identifying Target Populations: Predictive models are created to identify the patient populations most likely to meet the trial's inclusion criteria and have a higher probability of enrolling. This includes both general characteristics and specific criteria related to the trial. 3. Recruitment Source Optimization: Predictive analytics helps determine the most effective recruitment channels or sources for finding eligible participants. This can include targeted advertising, social media, healthcare providers, patient advocacy groups, and more. 4. Patient Engagement Insights: Predictive models can also provide insights into the factors that influence patient interest in clinical trials. By analyzing patient behaviors and preferences, CROs can tailor their recruitment strategies to better match patient expectations. 5. Screening Efficiency: Predictive analytics can improve the efficiency of patient screening. By accurately identifying potential candidates, trial sites can save time and resources, reducing the number of patients who do not meet the trial's criteria. |
Patient Retention Predictive Analytics: 1. Historical Data and Patient Behavior: For retention, predictive analytics considers historical data, as well as patient behavior and interactions throughout the trial. This may include appointment attendance, medication adherence, and study compliance. 2. Risk Identification: Predictive models are built to identify patients who are at risk of dropping out of the trial. Factors considered include missed appointments, non-adherence to the study protocol, and patient-reported issues. 3. Targeted Interventions: Once at-risk patients are identified, CROs can implement targeted interventions, such as personalized communication, reminder systems, additional support, or addressing specific patient concerns. 4. Resource Allocation: Predictive analytics helps CROs allocate resources more effectively by focusing efforts on patients who are most likely to benefit from interventions, rather than applying the same retention strategies to all participants. 5. Trial Adaptation: Based on predictive insights, CROs can make adjustments to the trial protocol, if feasible, to address issues that may contribute to patient dropouts. 6. Data-Driven Decision-Making: Predictive analytics in patient retention provides a data-driven approach to identifying issues early and proactively addressing them, ultimately improving patient engagement and study completion rates. |
Sash Barige
Dec/17/2021
Further Read:
Online resources covering the use of predictive analytics for patient recruitment and retention in clinical trials:
Predictive Analytics for Better Patient Recruitment and Retention (Clinpal): https://clinpalsolutions.com/patient-recruitment-retention-predictive-analytics/
Discusses how predictive analytics is applied for recruitment planning, identifying/targeting patients, optimizing enrollment, and improving patient retention.
Improving Clinical Trial Patient Recruitment and Retention with Predictive Analytics (Informa): https://www.informaconnect.com/improving-clinical-trial-patient-recruitment-and-retention-with-predictive-analytics/
Covers techniques like machine learning algorithms and AI to forecast patient enrollment, target recruitment, and reduce dropout rates.
Role of Predictive Analytics in Patient Recruitment and Retention (ResearchGate): https://www.researchgate.net/publication/342197088_Role_of_Predictive_Analytics_in_Patient_Recruitment_and_Retention
Academic paper examining predictive analytics approaches for patient recruitment forecasting, optimizing enrollment periods, and improving participant retention.
Applications of Predictive Analytics in Patient Recruitment and Retention (ClinCaptia): https://clincaptia.com/patient-recruitment-retention-predictive-analytics/
Reviews use cases and examples of using predictive analytics for enrollment planning, recruitment targeting, optimizing screen fail rates, and mitigating patient dropout.
Predictive Analytics: Improving Trial Patient Recruitment and Retention (PharmaPhorum): https://pharmaphorum.com/articles/predictive-analytics-improving-trial-patient-recruitment-and-retention/
Comments