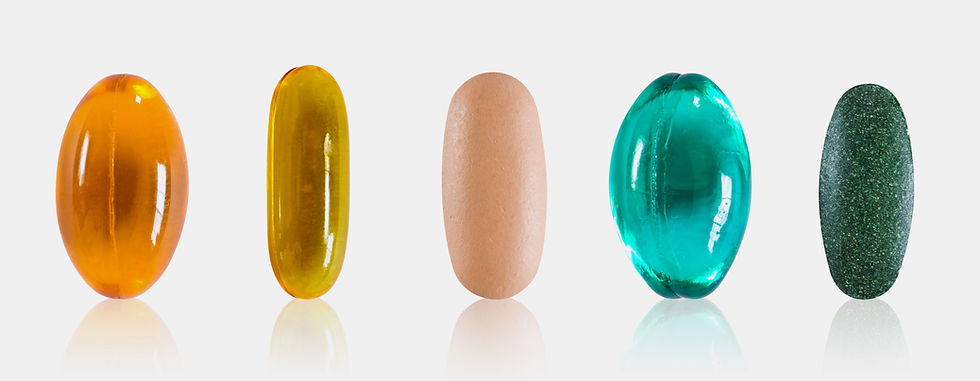
Predictive analytics used for patient randomization in clinical trials is a data-driven approach that helps researchers and clinical trial organizers optimize the allocation of participants to different treatment or intervention groups. The primary goal is to ensure that the randomization process is as unbiased and efficient as possible. Here's how predictive analytics is applied in patient randomization:
1. Patient Stratification:
2. Balance and Bias Reduction:
3. Adaptive Randomization:
4. Minimizing Predictable Patterns:
5. Treatment Effect Estimation:
6. Stratified Randomization:
7. Dynamic Allocation:
8. Maximizing Statistical Power:
9. Minimizing Ethical Concerns:
|
Start considering predictive analytics applications in trial planning, subject recruitment, site selection/optimization, and adaptive trial designs.
Sash Barige
Apr/17/2022
Further Read:
Patient Randomization and Clinical Trial Design Via Predictive Analytics (ResearchGate): https://www.researchgate.net/publication/269903605_Patient_Randomization_and_Clinical_Trial_Design_Via_Predictive_Analytics
This paper discusses using predictive analytics methods like regression and clustering for stratified patient randomization in clinical trials. It covers techniques to balance treatment arms.
Applications of Predictive Analytics in Improving Clinical Trials (PMTC): https://www.pmtc.org/blog/applications-predictive-analytics-improving-clinical-trials
This article covers using predictive analytics to select trial sites, identify eligible patients, optimize recruitment, and reduce patient dropouts. It provides real world examples.
Patient Randomization: From a Mathematician’s Perspective (Medium): https://medium.com/@sammytech17/patient-randomization-from-a-mathematicians-perspective-cdb6f8124c97
This post examines algorithms like block randomization and biased coin randomization for assigning patients to groups while preserving balance between arms.
Use of Predictive Analytics in Clinical Trials (IntelligencePharma): https://www.intelligencepharma.com/articles/predictive-analytics-in-clinical-trials
Comments