Power of Data Mesh: Use Cases
- Sash Barige
- Aug 20, 2022
- 3 min read
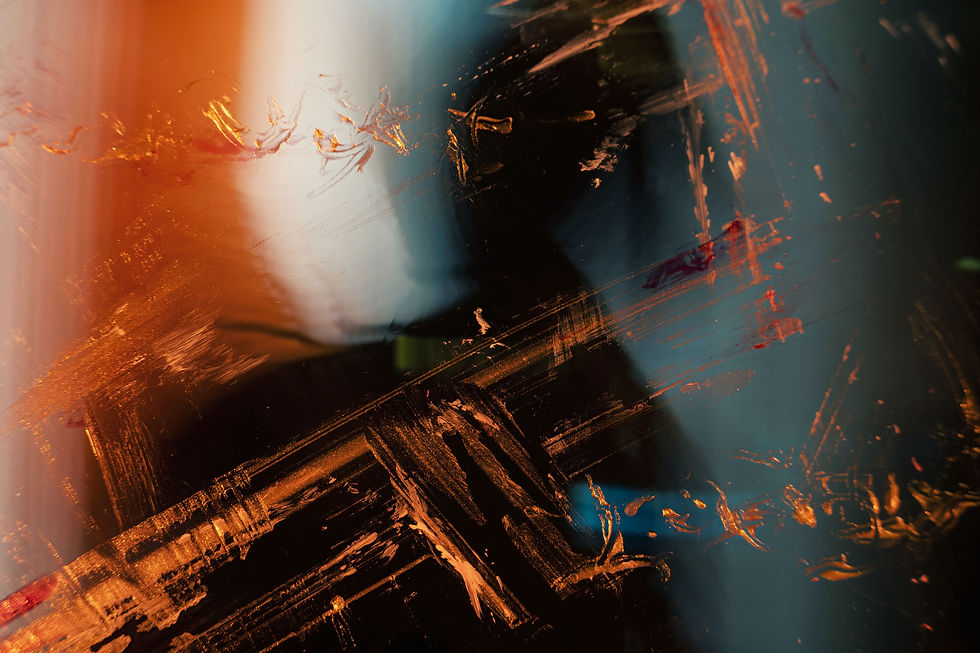
Don't Mesh with Data Mesh; I'm kidding, you may have use cases where data mesh might be appropriate!
In the ever-evolving world of data management, the concept of a "Data Mesh" has emerged as a groundbreaking approach to handling and scaling data in large, complex organizations. Coined by Zhamak Dehghani of ThoughtWorks in 2019, Data Mesh represents a paradigm shift in how we think about and manage data within modern enterprises. This article will explore what Data Mesh is, its use cases, companies implementing it, and the tools available to support its implementation.
Understanding Data Mesh
A Data Mesh is a decentralized and domain-oriented approach to data architecture that treats data as a product and leverages self-serve data infrastructure. Unlike traditional monolithic data architectures, Data Mesh divides the responsibility of data across different domains or "data products." Each domain or business unit within an organization owns and manages its own data, while cross-functional teams provide standardized data infrastructure and data governance services.
Use Cases for Data Mesh
Scalability and Complexity: Data Mesh is ideal for organizations dealing with vast and diverse datasets. It simplifies the process of managing and scaling data as it grows.
Cross-functional Collaboration: Large organizations with multiple business units and data consumers can benefit from Data Mesh, as it promotes collaboration and data sharing while maintaining data sovereignty.
Data Quality and Governance: Data Mesh encourages strong data governance practices, ensuring that data is standardized, high-quality, and compliant with regulations.
Data Monetization: Organizations looking to leverage their data as a strategic asset can use Data Mesh to create data products and monetize their information.
Companies Implementing Data Mesh
Several leading organizations have embraced the Data Mesh concept to improve their data management and analytics capabilities. Some prominent examples include:
LinkedIn: LinkedIn's data platform team adopted Data Mesh to address data scaling challenges, improve data discoverability, and foster cross-functional collaboration. They wanted to enable self-service access to data while maintaining data quality and governance.
Intuit: Intuit, a financial software company, embraced Data Mesh to create a more scalable and collaborative data architecture. They sought to break down data silos, improve data quality, and make data more accessible to different business units.
Spotify: Spotify adopted Data Mesh principles to better manage its extensive music streaming data. The goal was to enable different teams to work more independently and efficiently with data, ultimately improving their data-driven decision-making and product development.
WeWork: WeWork implemented Data Mesh to address issues related to data ownership and accessibility. By adopting Data Mesh, they aimed to empower cross-functional teams with self-serve data access while ensuring data quality and governance.
Walmart: Walmart, as one of the world's largest retailers, implemented Data Mesh to handle the massive amounts of data generated by its operations. Data Mesh allowed them to break down data silos and improve data quality, making data-driven decision-making more efficient.
Badoo (now Bumble): Badoo, a dating app company that is now part of Bumble, implemented Data Mesh to address data fragmentation and improve data discoverability. This approach allowed them to distribute data ownership to domain-specific teams and make data more accessible for analytics.
Cruise (GM Cruise LLC): GM Cruise LLC, a subsidiary of General Motors, adopted Data Mesh to manage the growing volume of data generated by autonomous vehicles. Data Mesh allowed them to scale their data infrastructure while maintaining data quality and governance.
Tools for Data Mesh
I'll write a separate post detailing many of the tools we've evaluated when considering if Data Mesh is appropriate for our architecture needs. Implementing a Data Mesh requires a set of tools that can support its principles. Some of the essential tools include:
DataHub: Developed by LinkedIn, DataHub is an open-source metadata search and discovery platform that helps organizations implement Data Mesh principles by providing a centralized catalog for data discovery.
LakeFS: LakeFS is an open-source platform that simplifies data lake management by introducing version control and branching capabilities, promoting data quality and governance.
Databand: Databand offers a data pipeline observability platform that assists in monitoring data pipelines, ensuring data quality, and providing visibility into data flow across the organization.
Great Expectations: Great Expectations is an open-source framework for data quality validation, documentation, and profiling, which can be instrumental in ensuring the quality and reliability of data products.
Data Mesh is a revolutionary approach to data architecture that has gained significant traction in recent years. With its focus on decentralization, scalability, and cross-functional collaboration, it is an attractive solution for large organizations facing complex data management challenges. As more companies adopt Data Mesh principles and develop supporting tools, it is likely to become a standard approach in the world of data management, enabling organizations to harness the true power of their data.
Sash Barige
Aug/20/2022
Photo: unsplash.com
References:
Dehghani, Z. (2019). Data Mesh: A Paradigm Shift in Data Platform Architecture. ThoughtWorks.
LinkedIn Engineering. (2022). DataHub: LinkedIn's Metadata Search and Discovery Platform. LinkedIn Engineering Blog.
Intuit. (2022). Embracing Data Mesh at Intuit. Intuit Engineering Blog.
Spotify Engineering. (2021). The Spotify Data Mesh. Spotify Engineering Blog.
LakeFS. (2022). Home. LakeFS.
Databand. (2022). Home. Databand.
Great Expectations. (2022). Home. Great Expectations.
https://martinfowler.com/articles/data-monolith-to-mesh.html
Komentar