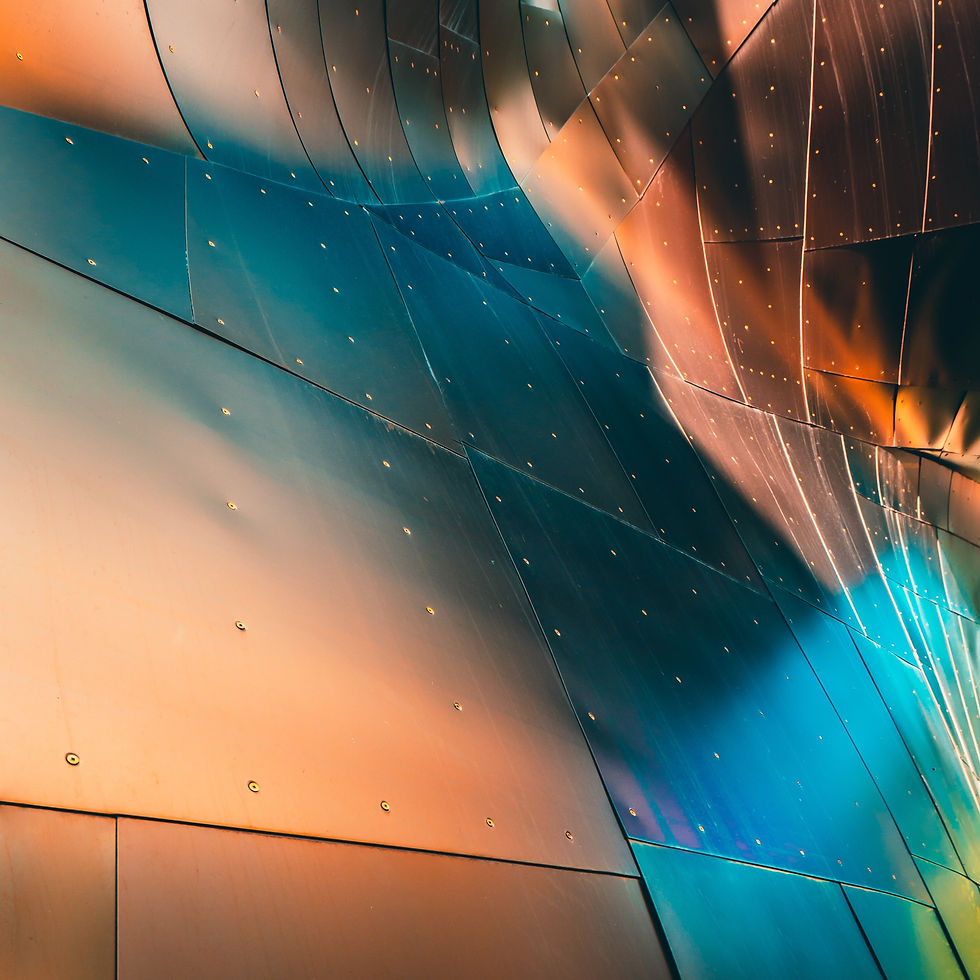
Snowflake and Databricks are both popular cloud-based data platforms, but they serve different purposes and excel in different areas. Here's a comparison of Snowflake and Databricks to help you understand their differences:
Snowflake:
Data Warehousing: Snowflake is primarily a cloud-based data warehousing platform. It's designed for storing and managing structured data, making it a good choice for analytical queries and reporting.
SQL-First Approach: Snowflake uses a SQL-first approach, which means it's particularly strong at handling structured data and performing complex SQL queries.
Data Integration: Snowflake provides features for data integration, transformation, and sharing, making it a suitable choice for data integration and analytics.
Performance: Snowflake is known for its excellent performance in handling large datasets for analytical workloads.
Scalability: Snowflake is scalable, allowing users to handle large datasets and scale their compute resources as needed.
Data Sharing: It offers features for easy data sharing and collaboration between different organizations or departments.
Databricks:
Unified Data Analytics: Databricks is a unified analytics platform that combines data engineering, data science, and machine learning in a single platform. It's more focused on data processing and data science.
Apache Spark: Databricks heavily relies on Apache Spark for distributed data processing, making it a strong choice for big data analytics and machine learning workloads.
Machine Learning: Databricks has built-in support for machine learning and deep learning, making it ideal for data science and AI projects.
Data Lake Integration: Databricks can seamlessly integrate with data lakes (e.g., Azure Data Lake Storage, AWS S3), allowing you to work with both structured and unstructured data.
Collaboration: Databricks provides collaborative tools and notebooks for data scientists and data engineers to work together on projects.
Real-Time Processing: Databricks supports real-time data streaming and processing, making it suitable for real-time analytics use cases.
Flexibility: Databricks is highly flexible, allowing users to work with a variety of data sources and languages, including Python, R, and Scala.
In summary, the choice between Snowflake and Databricks depends on your specific needs and use cases. If you primarily need a data warehousing solution for structured data and complex SQL queries, Snowflake is a strong choice. If you are focused on data engineering, data science, big data analytics, and machine learning, Databricks is a more suitable option. In some cases, organizations may choose to use both platforms in conjunction to cover a wider range of data processing and analytics needs.
Sash Barige
Sep/05/2021
Photo Credit: Unsplash.com
Comments