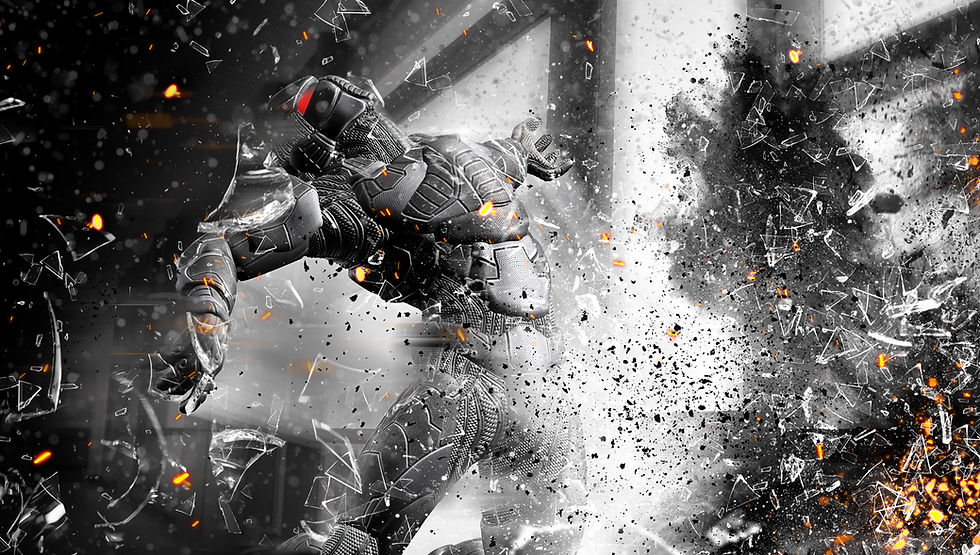
Master Data Management (MDM) can be used within a Data Mesh, but the approach to MDM within a Data Mesh differs from traditional MDM practices. In a Data Mesh, data ownership is distributed across different domain teams, making it essential to adapt MDM to this decentralized and domain-oriented model. Here's how you can incorporate MDM principles within a Data Mesh:
Define Data Domains for MDM: Identify specific data domains where master data is critical. This could include customer data, product data, employee data, and other core entities that need to be consistent and well-maintained across the organization. | Domain Ownership: Assign ownership of master data domains to specific domain teams within the Data Mesh. Each domain team is responsible for the quality, governance, and maintenance of the master data within their domain. | Standardize MDM Processes: Establish standardized MDM processes, such as data quality checks, data validation rules, and data stewardship responsibilities for each domain. These processes should align with the principles of the Data Mesh. | Metadata and Data Catalog: Ensure that metadata and data catalog tools within the Data Mesh capture information about master data entities, their definitions, and data lineage. This metadata is essential for tracking and understanding master data. |
Data Quality and Validation: Implement data quality frameworks and validation checks, such as Great Expectations, to ensure that master data meets predefined expectations and quality standards. Data product teams should incorporate these checks into their processes. | Data Access APIs: Define APIs for accessing master data within each domain. These APIs should provide controlled and standardized access to master data, promoting self-service while maintaining data quality. | Data Governance: Create a clear data governance framework for master data, including data ownership, data stewardship roles, and compliance with regulatory requirements. | Data Synchronization: When necessary, set up mechanisms for data synchronization or data sharing between domains that require access to the same master data. This should be done in a way that respects the autonomy and ownership of each domain. |
Collaboration and Communication: Encourage collaboration and communication between domain teams, particularly when changes to master data are needed. Domain teams should collaborate on changes and ensure that they are applied consistently across the organization. | Version Control for MDM Processes: Implement version control for MDM processes and workflows. This ensures that changes to master data are tracked, documented, and reproducible. | Data Observability and Monitoring: Continuously monitor the quality and usage of master data within the Data Mesh, using data observability tools to ensure that it remains consistent and reliable. | Feedback Loop: Establish a feedback loop between domain teams and the central MDM team (if one exists) to provide input, address issues, and iterate on MDM processes. |
Sash Barige
Sept/10/2022
Photo credit: unsplash.com
Building an Event-Driven Data Mesh: https://www.oreilly.com/library/view/building-an-event-driven/9781098127596/ by Adam Bellemare
Data Mesh: The Decentralized Data Architecture That's Revolutionizing Data Management: https://towardsdatascience.com/data-mesh-the-balancing-act-of-centralization-and-decentralization-f5dc0bb54bcf by Forbes
Data Mesh: A New Paradigm for Data Management: https://siliconangle.com/2021/10/27/data-mesh-new-paradigm-data-management/ by Gartner
Data Mesh: The Future of Data Management: https://medium.com/@danilo.drobac/data-mesh-the-future-of-data-modeling-and-scalable-analytics-57670a460a47 by McKinsey & Company
Data Mesh: The Next Big Thing in Data Management: https://revelate.co/blog/data-mesh/ by The Data Mesh Alliance
Kommentare