Data Governance Step by Step: Month 2 Week 1
- Sash Barige
- Apr 30, 2022
- 1 min read
Updated: Nov 3, 2023
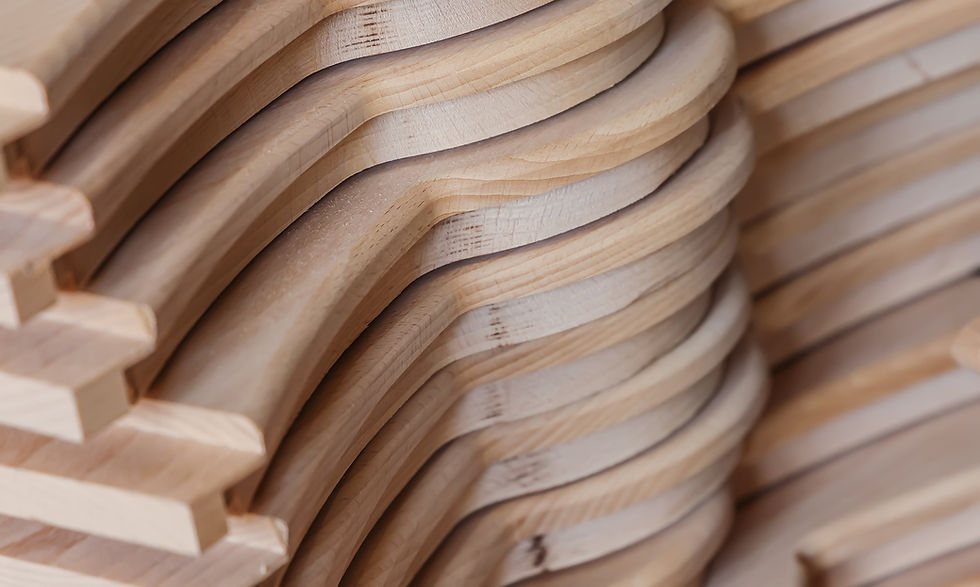
Month 2:
Identify key data assets and systems: Which data assets are most critical to your business? Which systems are used to collect, store, and manage these data assets?
Data Quality Assessment: Evaluate the quality of your data to identify issues like inaccuracies, duplication, and inconsistency. This will be impactful to get buy-in from the executive committee.
Identify Project Management Approach: Do you have a PMO (project management office)? Assess project management process used and specific project 'gates' that gates data activities. Identify gaps.
Categorize Business Needs and Key Challenges: Based on the surveys and assessments conducted during the previous month, build out use case priority and impact matrix.
Establish a data governance framework: This framework should define the roles and responsibilities of key stakeholders, as well as the policies and procedures that will govern the use and management of data.
Define Data Governance Roles & Responsibilities
Define the scope and goals of your data governance program: What do you want to achieve with data governance? Do you want to improve data quality, enhance compliance, or optimize data utilization? Once you know your goals, you can tailor your implementation plan accordingly. What problems are you trying to solve, and what benefits do you expect to gain? Set clear objectives by defining the goals and objectives of your data governance program. Note, this is at a draft stage at this point. You'll need to review with the governance steering committee to finalize.
Data Governance Charter: Develop a draft data governance charter, stakeholder map and policy outline. Review it by the leadership influencers.
Data Catalog: Begin developing a data dictionary/catalog.
Identify members for Executive Steering Committee
Week 1
Identify key data assets and systems: Which data assets are most critical to your business? Which systems are used to collect, store, and manage these data assets? In the previous month, during the data influencer surveys and data architecture assessment, we'll have a good understanding of they key data assets and the key systems. This determination should be based on the business team assessment and preferably not the IT team's assessment. In terms of system, you'll identify core business systems, core operational systems, core corporate level systems (e.g. ERP) that the business would rank them higher than the IT infrastructure, IT middleware etc. |
I'll share examples in different industries to give you an idea of the level
Key data assets and systems in a clinical research organization (CRO) include:
In addition to these systems, CROs may also use a variety of other systems, such as project management systems, quality management systems, and financial management systems. |
Key data assets and systems in an Electronic Medical Record (EMR) healthcare IT organization include:
Data assets:
Systems:
In addition to these systems, EMR healthcare IT organizations may also use a variety of other systems, such as revenue cycle management systems, patient portals, and interoperability solutions. |
Key data assets and systems in a financial services organization include:
Data assets:
Systems:
In addition to these systems, financial services organizations may also use a variety of other systems, such as trading platforms, portfolio management systems, and compliance systems. |
Data Quality Assessment: Evaluate the quality of your data to identify issues like inaccuracies, duplication, and inconsistency. This will be impactful to get buy-in from the executive committee. I managed a technical team and prioritized them to perform ad-hoc analysis of the data. I had previous experience using TAMR and provisioned it for data quality analysis. TAMR uses machine learning capabilities to perform massive data comparisons to identify data duplicates and other data quality issues. If I get time, I'll do a separate post on TAMR and how to leverage it (or similar tools) for these needs. Note, I also used Informatica tools (Informatica Data Quality) for data quality analysis. However this was not during Month 2 Week 1. In my previous company, I'd done similar analysis using Talend. The presentation materials I'd created had charts illustrating the extent (quantify) of quality issues and explained (qualified) how this will impact loss of revenue, loss of trust in data, etc. |
Sash Barige
Apr/30/2022
Comments